Sci-Net
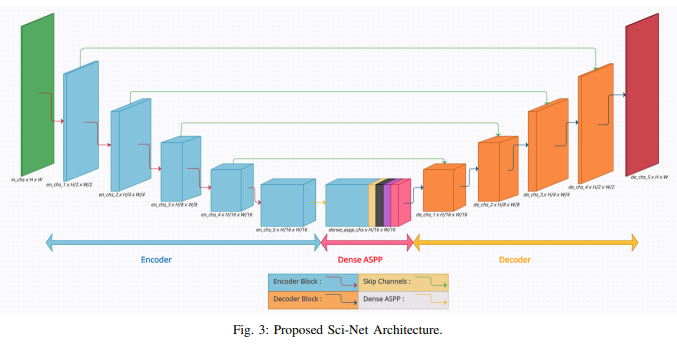
Buildings' segmentation is a fundamental task in the field of earth observation and aerial imagery analysis. Most existing deep learning based algorithms in the literature can be applied to fixed or narrow-ranged spatial resolution imagery. In practical scenarios, users deal with a wide spectrum of images resolution and thus, often need to resample a given aerial image to match the spatial resolution of the dataset used to train the deep learning model. This however, would result in a severe degradation in the quality of the output segmentation masks. To deal with this issue, we propose in this research a Scale-invariant neural network (Sci-Net) that is able to segment buildings present in aerial images at different spatial resolutions. Specifically, we modified the U-Net architecture and fused it with dense Atrous Spatial Pyramid Pooling (ASPP) to extract fine-grained multi-scale representations. We compared the performance of our proposed model against several state-of-the-art models on the Open Cities AI dataset, and showed that Sci-Net provides a steady improvement margin in performance across all resolutions available in the dataset.
Paper preprint can be fetched here.